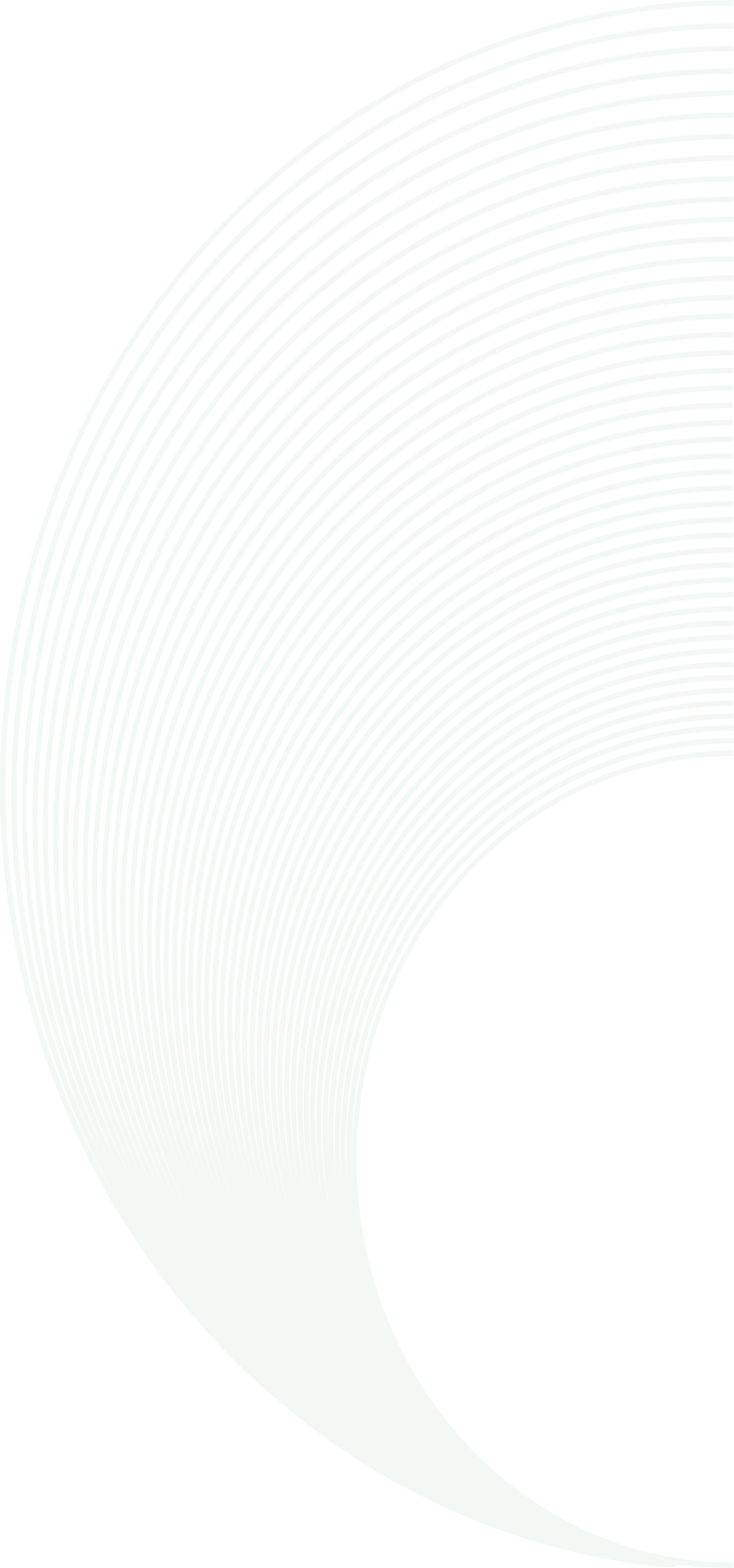
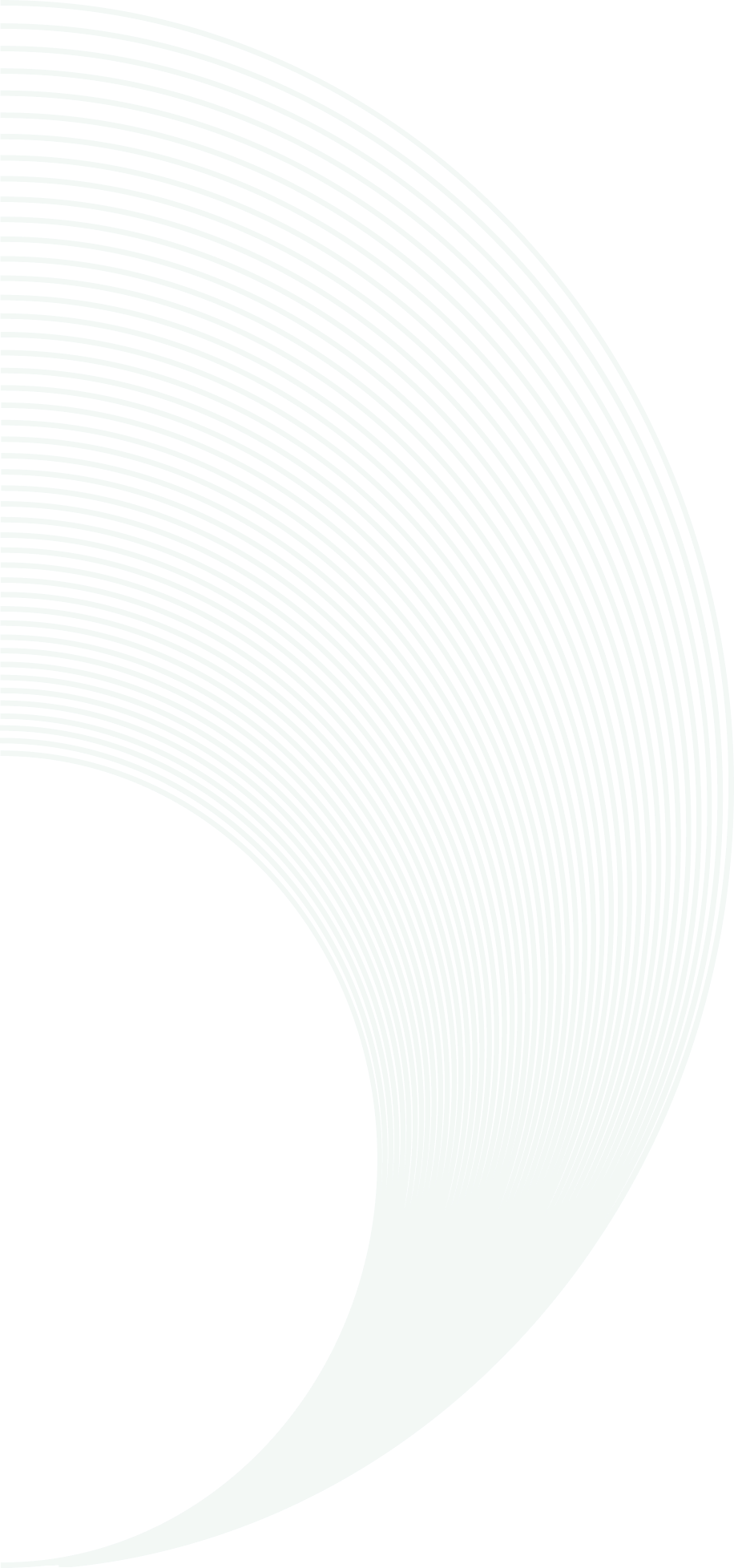
The Future of Lab Automation: Emerging Technologies and Trends
Discover the future of lab automation with emerging technologies and trends revolutionizing research, diagnostics, and efficiency in laboratory workflows.
The world of lab research is changing faster than ever. Efficiency, precision, and innovation are taking center stage, and all these have increasingly important backing from automation. Today, powerful technologies like AI, ML, and IoT are gradually gaining acceptance in labs researching biotechnology, pharmaceuticals, and chemicals. Besides making the performance of labs smoother, they are drastically changing the ways of conducting experiments and data interpretation. As these technologies increase in adoption for incorporation in reactor design and lab operations, we will be dealing with labs that are unprecedentedly connected, data-driven, and efficient.
Lab Automation by Artificial Intelligence
AI is at the helm of lab automation innovations, where the processing and interpretation of data by researchers are going through a revolution. AI minimizes manual labour, expedites workflows, and generates more accurate outcomes by letting computers analyze complex datasets and identify patterns.
AI in lab reactors can significantly enhance efficiency by optimizing conditions in real-time. For example, an AI-controlled lab reactor can alter temperature, pressure, and mixing speeds based on constant monitoring so that ideal conditions for every experiment will be provided without human interference. AI algorithms can further assist in designing better experiments as they evaluate millions of parameters and then find the one most likely to produce desirable results. This allows for hypothesis testing that is quicker and more accurate, and therefore researchers can save ample time and resources.
Lab Data Analysis using Machine Learning (ML)
It has been the case that applications like machine learning apply AI where systems can learn and evolve from experience, rather than being explicitly programmed. While in the lab, this ML improves the processing of data to an extent, so automation systems can deduce insights from huge heaps of data much better. Its difference from conventional analysis tools is that an ML model can detect a pattern as well as a relationship between things that might go unnoticed under traditional analysis techniques, making insights into experimental results much more profound for the researchers involved.
Internet of Things and Connected Laboratories
The Internet of Things is transforming laboratories into interconnected settings where equipment, sensors, and systems can communicate with one another seamlessly. IoT in a lab setting allows the sharing of real-time data, remote monitoring, and better collaboration from different research teams. This comes in handy in large labs with several experiments running in parallel, as IoT permits researchers to track and regulate experiments from any location.
In lab reactors, IoT-enabled sensors continuously gather data on temperature, pressure, pH levels, and other critical parameters to forward the information to the central systems where it would be monitored and analyzed. The combination of IoT with AI and ML brings the concept of "closed-loop automation" to labs. Closed-loop simply refers to systems wherein the output of IoT sensors provides critical information to AI and ML algorithms that analyze such inputs and make real-time variations in reactor settings to come up with a fully automated, self-regulated ecosystem. The outcome is one such system where consistency between all experiments increases, the levels of downtime decrease, and lab productivity increases.
Evolution in Lab Reactors through Design Revolution through Automation
In other words, the lab reactor is the core of most chemical, pharmaceutical, and biotechnological research. AI, ML, and IoT integration have just started to transform the reactor into something adaptive, efficient, and versatile. Modern reactor design with high-end sensors and AI algorithms makes an automatic adaptation to optimum experimental conditions possible, giving this modern reactor a precision control better than that in a traditionally manually adjusted reactor.
Another is the modular reactor design which allows for interchangeable components to be designed. These modules could be rearranged to achieve specific conditions in an experiment, thereby making reactors versatile and cheaper
The emerging technologies in the form of AI, ML, and IoT have many benefits that come from an enhancement in productivity, higher accuracy, better insights from data, and even a saving of resources in experiments through automation. AI, ML, and IoT would increase the precision in experimental conditions, reduce manual intervention in lab automation, and eventually pave the way for better, reliable, and reproducible results in experiments. With IoT providing real-time feedback, AI and ML capabilities will further enhance the ability for continuous process improvements that are highly critical in long-term research and development.
However, there are several disadvantages to the implementation of these technologies. The high-tech lab equipment is quite expensive for smaller labs to acquire, and using automated systems takes some time to train personnel in terms of how to use the equipment and interpret data coming from the automated system. Data security is another very big issue, especially when considering IoT-enabled devices that will continuously transmit information. This calls for robust cybersecurity mechanisms to protect sensitive research data from unauthorized access.
The future of lab automation is being shaped by transformative technologies such as AI, ML, and IoT, changing how laboratories operate, experiment, and innovate. It brings increased efficiency, accuracy, and data-driven decision-making capabilities to labs that no one has ever seen before. While challenges still abound, the benefits are clear, and embracing these trends will probably be the way forward to achieve and stay at the competitive edge of scientific research and development.